Harnessing Predictive Analytics: Transforming Business Forecasting
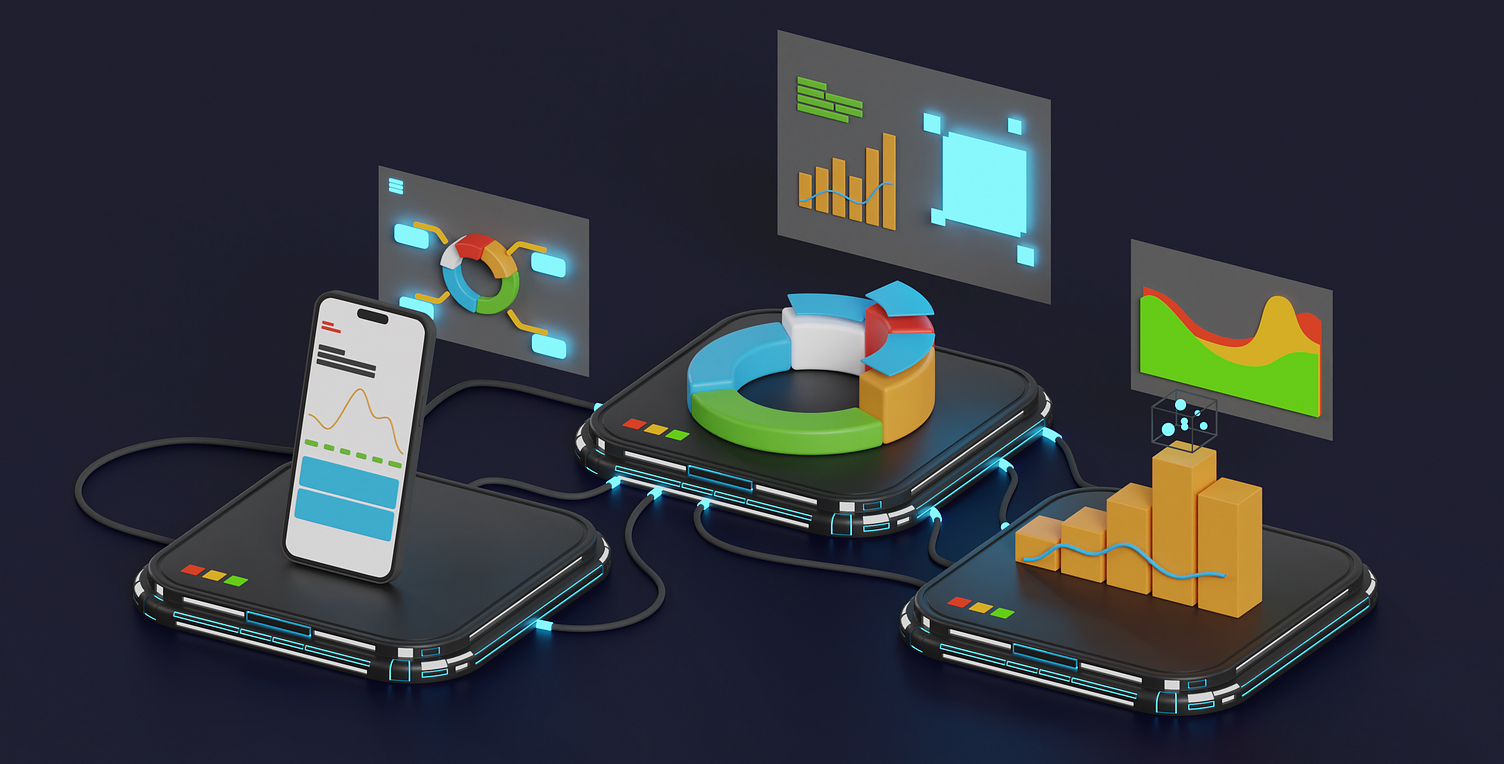
Predictive analytics is a powerful tool that uses historical data, statistical algorithms, and machine learning techniques to identify the likelihood of future outcomes. By leveraging predictive analytics, businesses can anticipate trends, make proactive decisions, and optimize operations. This blog delves into the evolution of predictive analytics, its applications across various industries, and the promising future trends that are shaping the landscape.
The Evolution of Predictive Analytics
Predictive analytics has come a long way from its early days of simple linear regression models. Initially, businesses used basic statistical methods to forecast future trends based on historical data. However, as data volumes grew and computing power increased, the field expanded to include a wide array of advanced techniques capable of handling complex and large datasets.
Today, predictive analytics incorporates sophisticated machine learning algorithms and AI technologies. These advancements enable businesses to uncover deeper insights, identify patterns that were previously hidden, and make more accurate predictions. The evolution of predictive analytics reflects the growing importance of data-driven decision-making in the modern business environment.
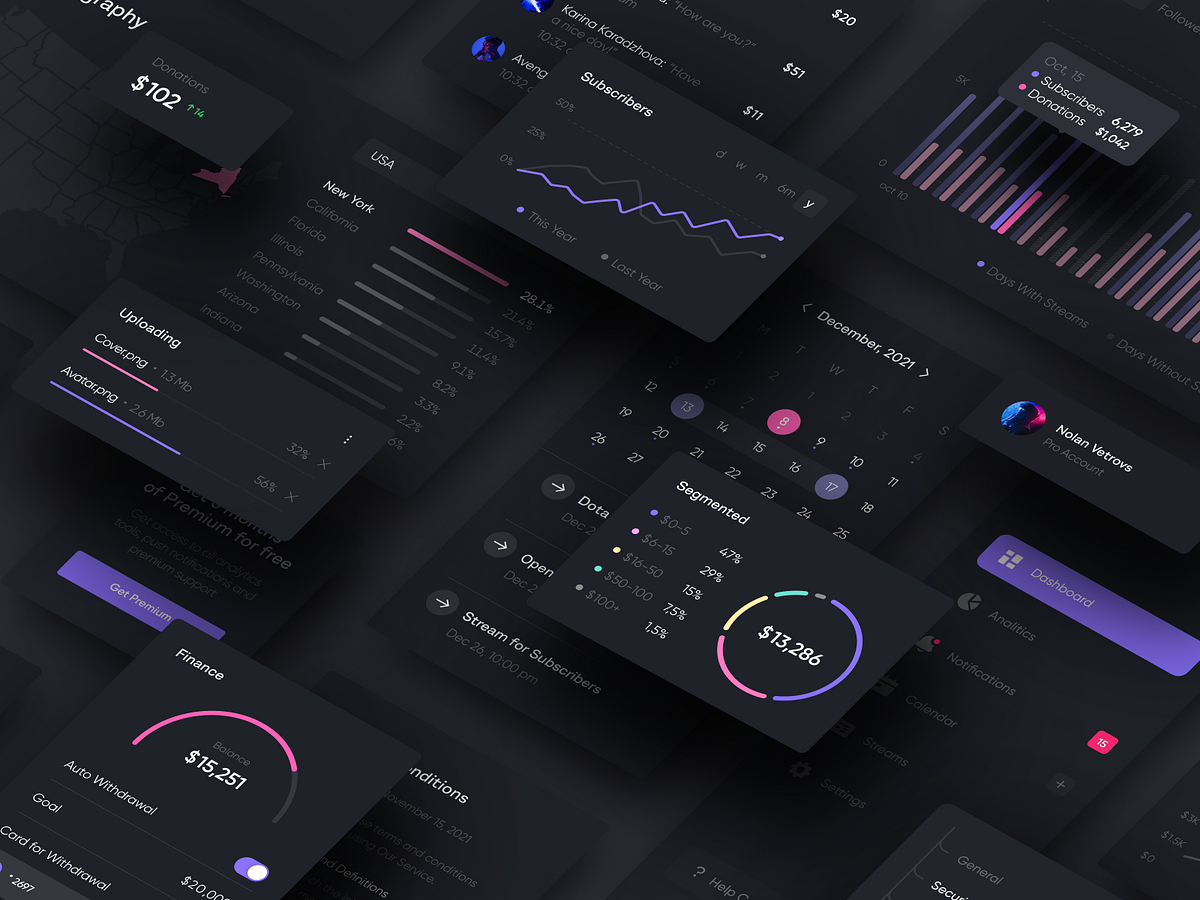
Applications in Various Industries
Predictive analytics has transformative potential across multiple industries, each reaping significant benefits from its implementation:
Finance
In the finance sector, predictive analytics is used to forecast stock prices, assess credit risk, and detect fraudulent activities. Financial institutions leverage these insights to make informed investment decisions, mitigate risks, and enhance security measures. For instance, by analyzing transaction patterns, banks can identify unusual activities that may indicate fraud, allowing them to take preventive action promptly.
Healthcare
The healthcare industry uses predictive analytics to forecast patient admissions, personalize treatment plans, and predict disease outbreaks. Hospitals and clinics can optimize their resources and improve patient care by anticipating surges in patient numbers or identifying individuals at high risk of certain conditions. Personalized treatment plans based on predictive insights lead to better patient outcomes and more efficient healthcare delivery.
Retail
Retailers utilize predictive analytics to optimize inventory management, personalize marketing campaigns, and forecast sales trends. By analyzing consumer behavior and purchasing patterns, retailers can ensure they have the right products in stock, target customers with relevant promotions, and maximize sales opportunities. For example, predictive analytics can help retailers determine which products are likely to be in high demand during specific seasons, allowing them to adjust their inventory accordingly.
Manufacturing
In the manufacturing sector, predictive analytics helps predict equipment failures, optimize maintenance schedules, and improve supply chain efficiency. By monitoring machinery and analyzing performance data, manufacturers can predict when equipment is likely to fail and schedule maintenance before breakdowns occur. This proactive approach reduces downtime, extends the lifespan of machinery, and enhances overall operational efficiency.
Key Capabilities of Predictive Analytics Solutions
Predictive analytics solutions offer a range of capabilities that empower organizations to transform their data into actionable insights. Key features include:
Data Integration
Effective predictive analytics requires seamless integration of data from various sources. By combining information from different departments, systems, and external databases, organizations gain a comprehensive view of their operations. This holistic approach ensures that predictions are based on complete and accurate data, leading to more reliable outcomes.
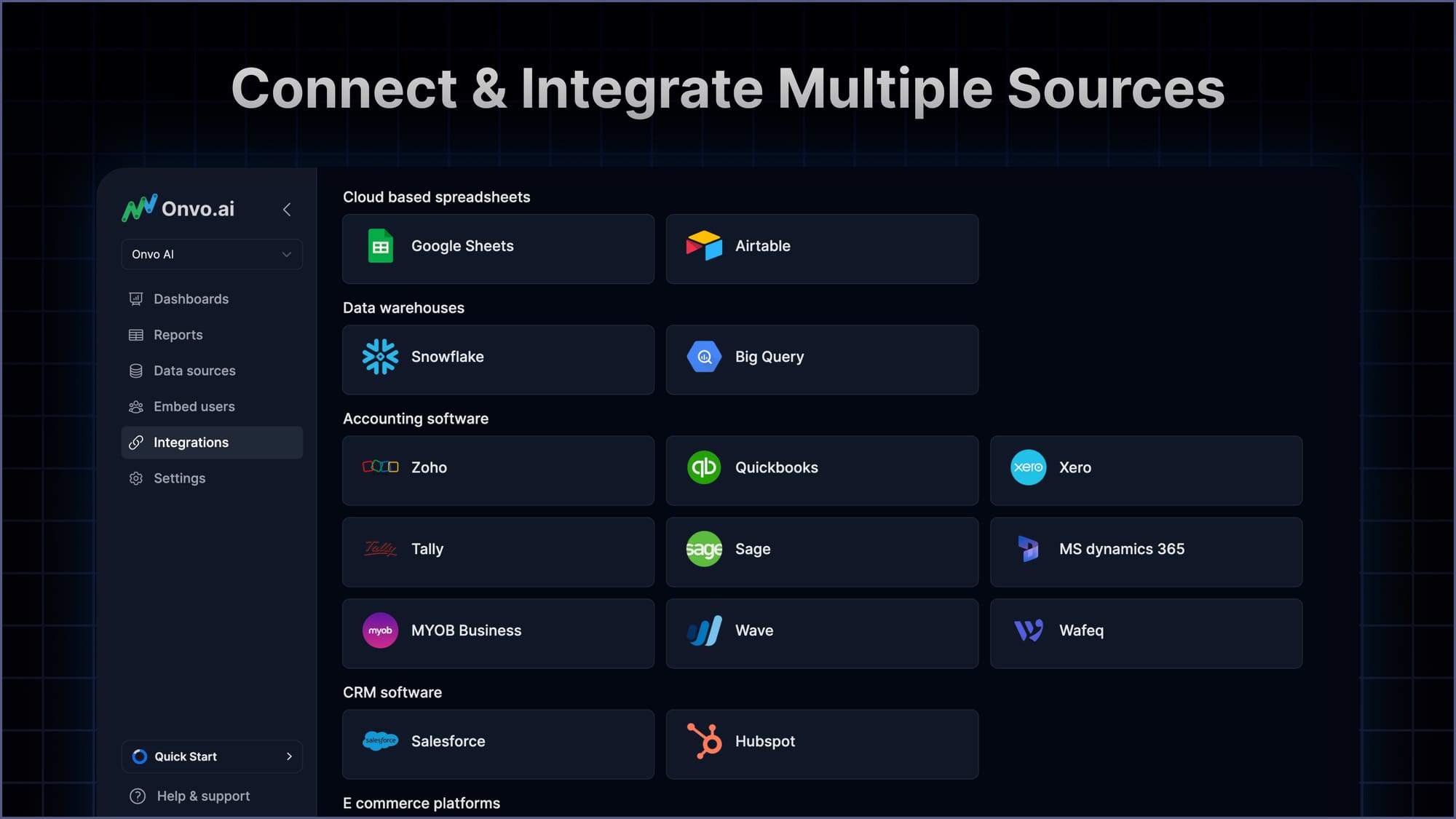
Advanced Modeling
Advanced modeling techniques, including machine learning and statistical models, enable businesses to predict future trends and behaviors with high accuracy. These models analyze historical data to identify patterns and correlations, providing valuable insights into what is likely to happen next. For example, a retail business can use advanced modeling to predict future sales based on past trends, helping them plan their inventory and marketing strategies effectively.
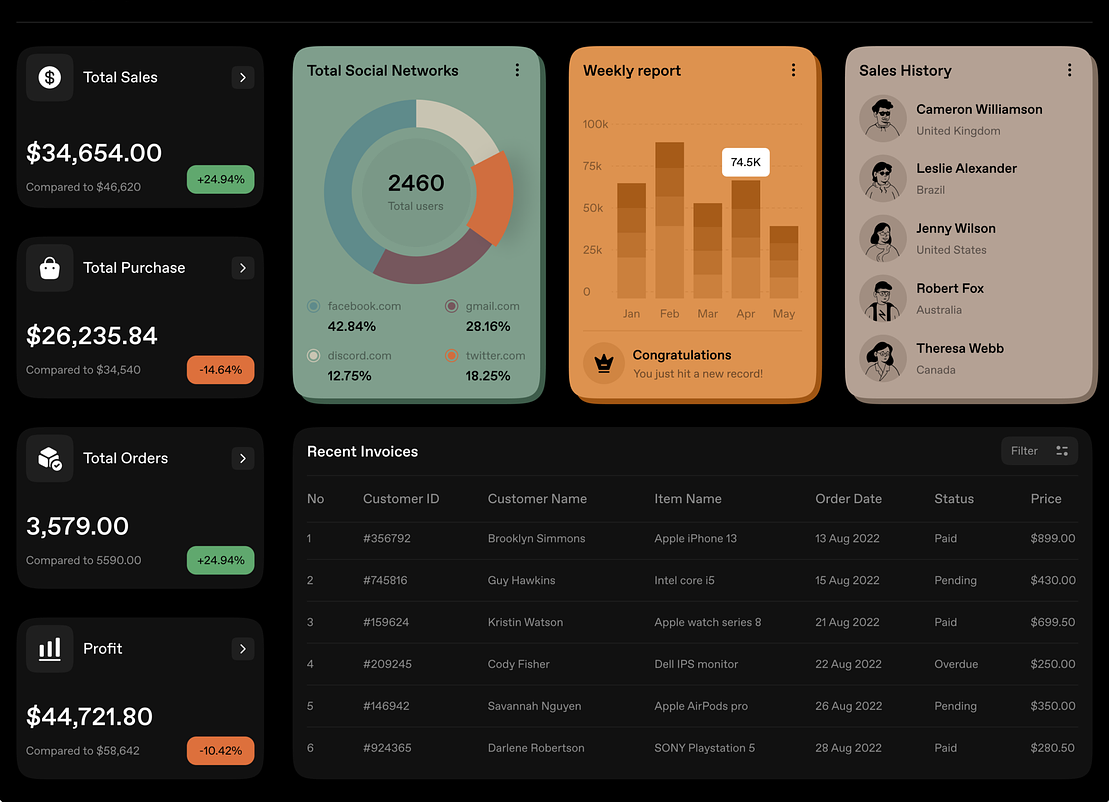
Real-Time Analytics
Real-time analytics capabilities allow organizations to generate predictions on the fly, supporting immediate decision-making. This is particularly valuable in fast-paced industries where timely insights can make a significant difference. For instance, in the finance sector, real-time analytics can provide instant alerts about market shifts, enabling traders to react quickly and make profitable decisions.
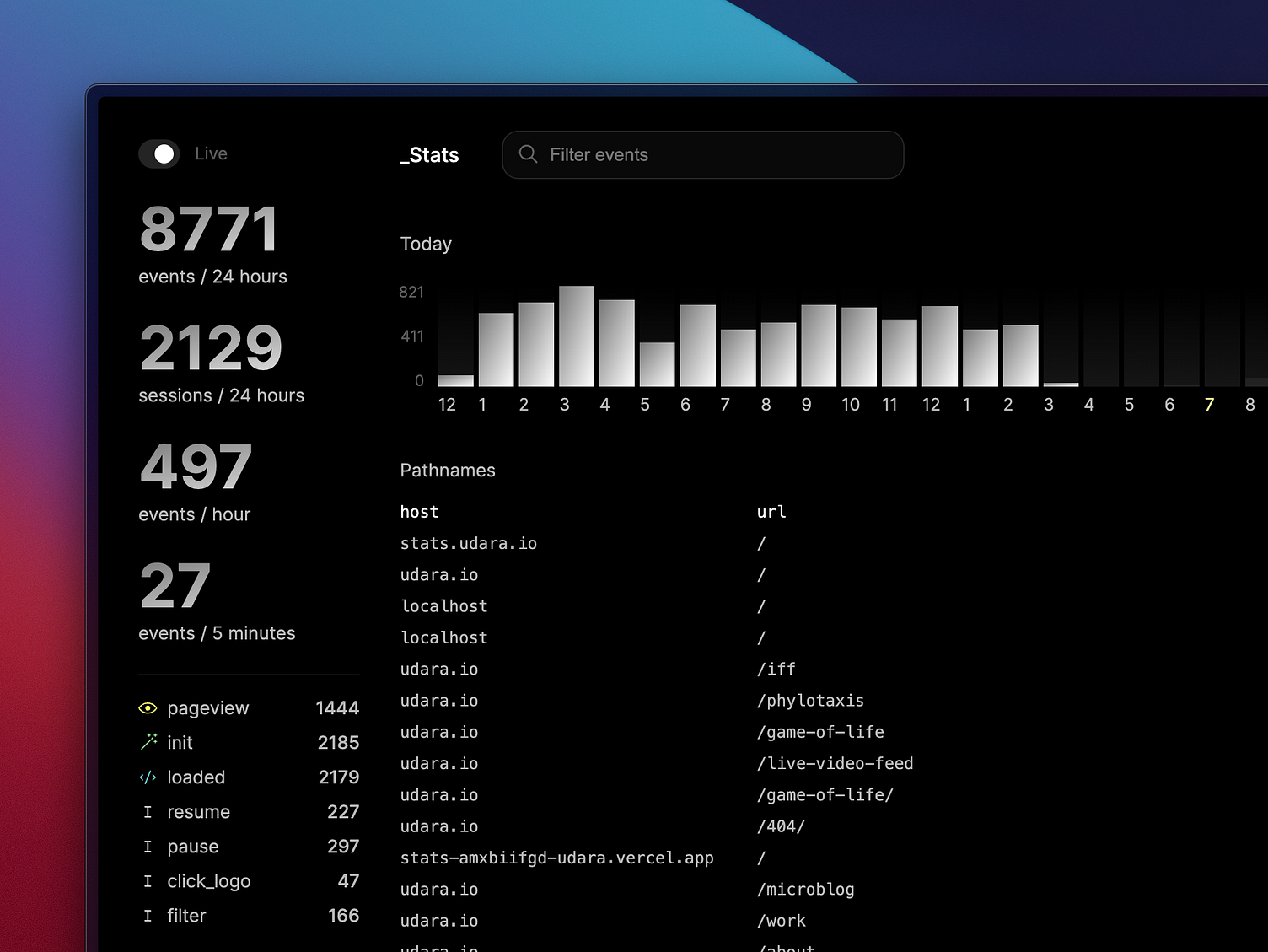
Customizable Dashboards
Interactive dashboards offer a user-friendly interface for exploring predictions and scenarios. Businesses can customize these dashboards to display the most relevant metrics and KPIs, making it easier to monitor performance and make informed decisions. For example, a sales manager can use a dashboard to track sales forecasts and compare them with actual performance, identifying areas that need attention.
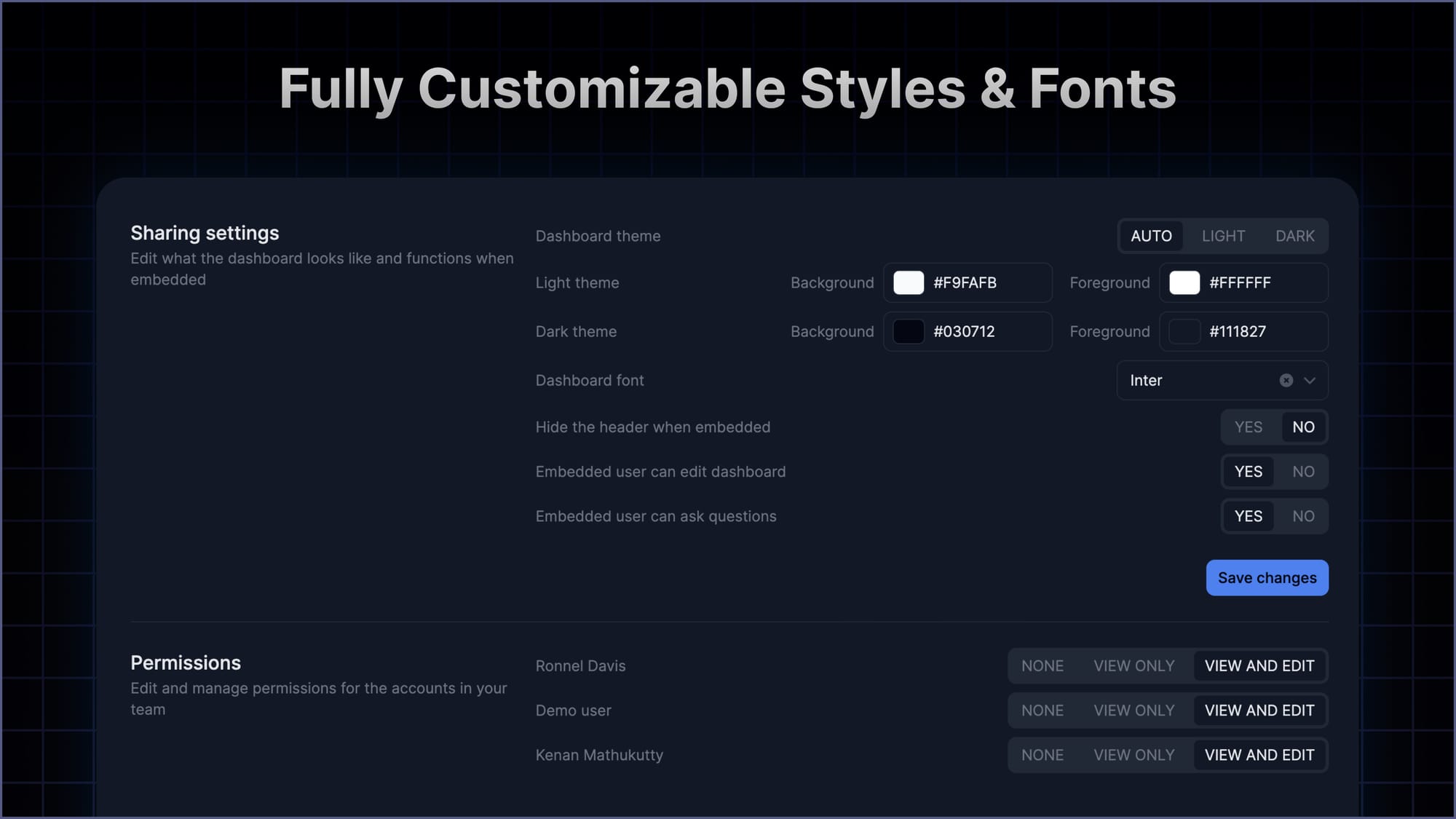
Future Trends in Predictive Analytics
The future of predictive analytics is promising, with several emerging trends set to enhance its capabilities further:
Automated Machine Learning (AutoML)
Automated Machine Learning (AutoML) simplifies the process of building and deploying predictive models. AutoML platforms automate many of the complex tasks involved in model development, making predictive analytics more accessible to businesses without extensive data science expertise. This democratization of predictive analytics enables more organizations to harness its power and drive innovation.
Explainable AI
Explainable AI is a growing trend aimed at enhancing transparency and trust in predictive models. By making the decisions of AI algorithms interpretable, businesses can understand how predictions are made and ensure they align with their objectives. This transparency is particularly important in regulated industries, such as finance and healthcare, where accountability and compliance are critical.
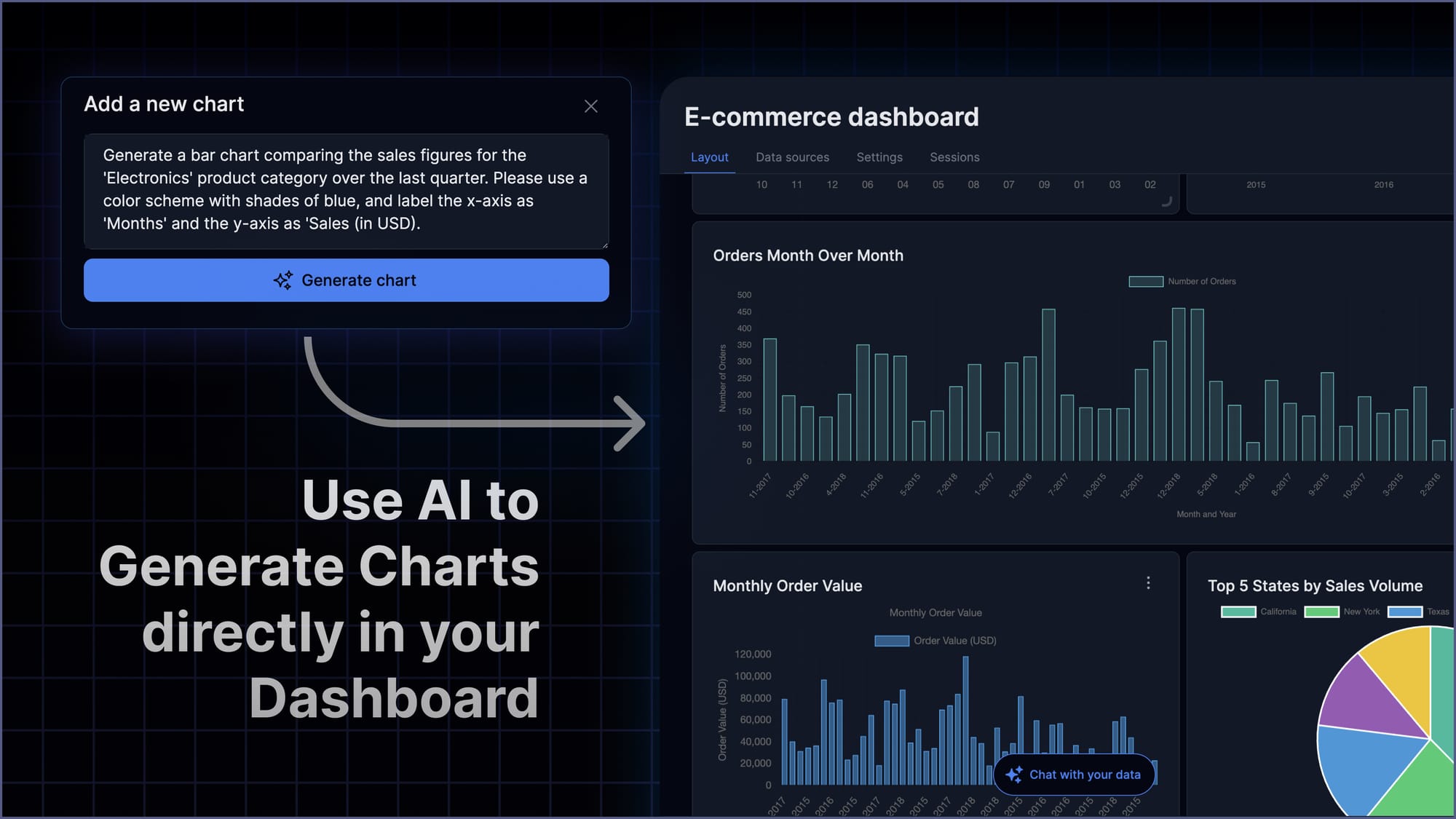
Edge Analytics
Edge analytics involves performing predictive analytics closer to where data is generated, rather than relying solely on centralized data centers. This approach reduces latency and enables faster insights, making it ideal for applications that require real-time decision-making. For example, in manufacturing, edge analytics can analyze data from sensors on production lines to predict equipment failures and trigger immediate maintenance actions.
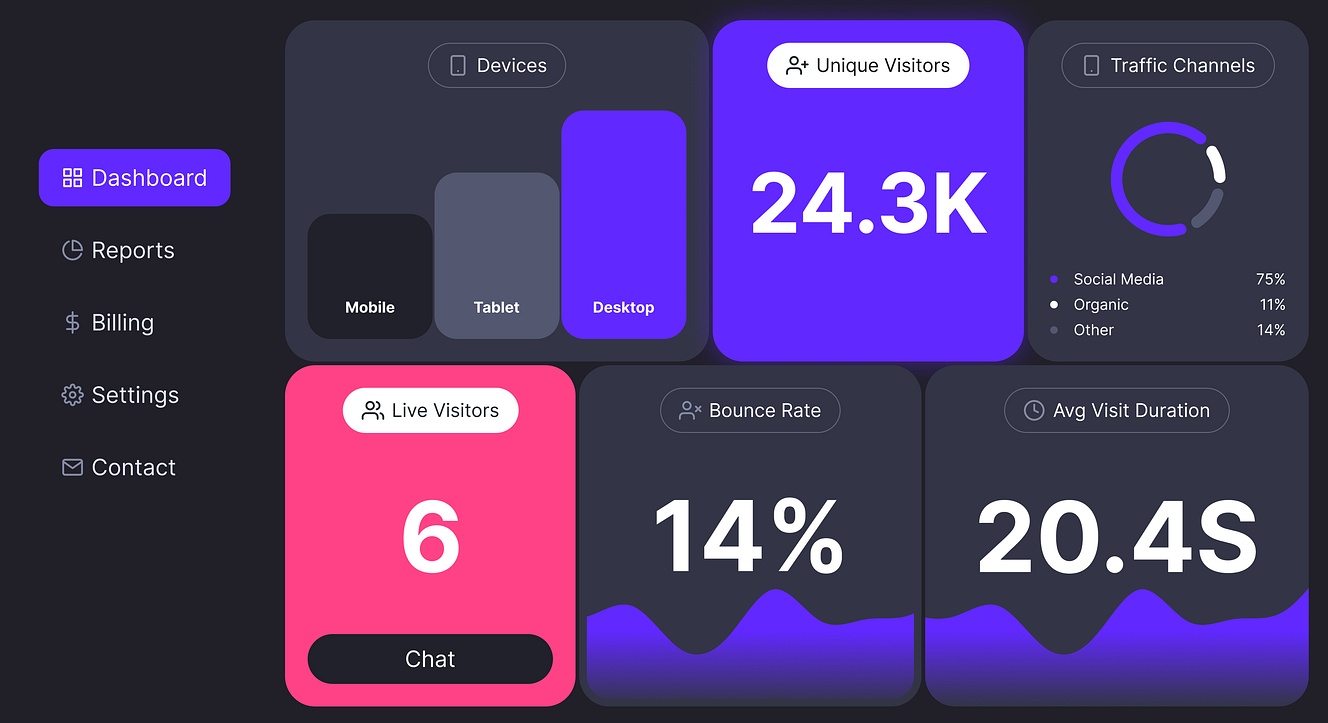
Conclusion
Predictive analytics is revolutionizing the way businesses operate, providing the foresight needed to make strategic decisions. By leveraging historical data, advanced modeling techniques, and real-time analytics, organizations can anticipate trends, optimize operations, and stay ahead of the competition. As the field continues to evolve, emerging trends like AutoML, explainable AI, and edge analytics will further enhance its capabilities, making predictive analytics an indispensable tool for modern businesses.
Embrace the power of predictive analytics to transform your business forecasting and drive long-term success. Stay tuned for more insights and updates from the world of data-driven decision-making.
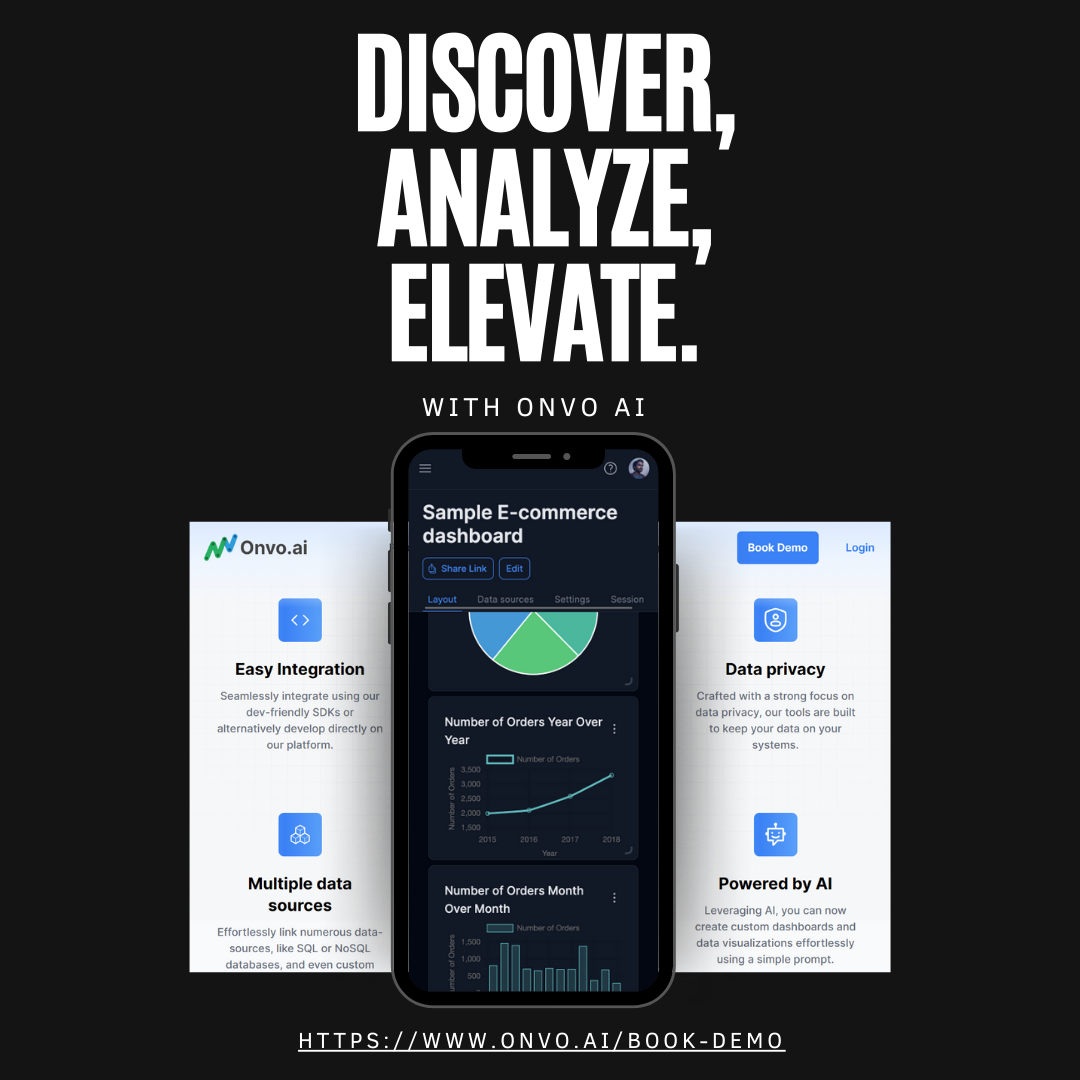
Stay tuned for more insights and updates from the world of data-driven decision-making with Onvo.